Regression Analysis
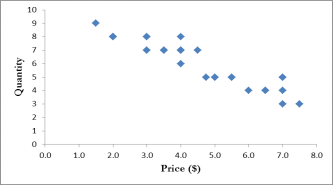
There are broadly two ways in which we can think about quantifying data:
- Making predictions one period ahead.
- Making predictions more than two periods ahead.
This is done through regression analysis.
Regression is about quantifying the relationship between two or more variables.
Example: Suppose you're looking at demand or data of people purchasing, and you know how prices were changing. What you'd like to do is to think about how you can start thinking about how price is changing demand.
What we are trying to do is to explain a dependent variable (sales or demand), as a function of independent variables (price).
- With regression we try to make predictions of what would be the demand at different prices.
- Regression is a technique that uses simple linear additive model to make these kinds of predictions.
Example: Demand Analysis
What this firm was trying to do is to try and understand how their prices might change demand. So they changed the prices and then observed the demand.
Price ($)
Demand
4.0
7
3.5
7
5.0
5
6.0
4
6.5
4
7.0
4
2.0
8
4.0
6
5.5
5
3.0
7
3.5
7
2.0
8
2.0
8
3.0
8
3.0
7
1.5
9
3.0
8
4.8
5
5.0
5
4.0
7
4.5
7
4.0
8
7.5
3
4.0
7
6.5
4
4.0
7
7.0
3
5.5
5
7.0
5
3.5
7
7.0
5
2.0
8
We see that as prices go up, the demand goes down.
What regression helps with here, is that it gives specific numbers. With regression, we can tell by how much the sales will reduce for a certain increase in price.
Question answered: If I increase price by 1 $, by how much does the sales come down?
Demand Analysis
Salest = a + b1 Pricet + et
Here,
- Sales is the dependent variable
- Price is the independent variable.
- b = price sensitivity à captures how sensitive your demand is to price
- a = intercept à captures the baseline level of demand
- e = error term à captures the increase/decrease in demand due to factors other than price (For example, they could be promotions, advertising, competitive actions, and so on)
Simple Regression:
Yt = a + b1 * X1t + et
This is the more general form of what we discussed above. This is termed as simple regression. The idea here is that the dependent variable, y, which is on the left-hand side, is related to the independent variable, x, which is on the right-hand side.
How does regression work?
Regression tries to fit a straight line, in this case, the demand equation, to the data that we have.
The regression line is downward sloping à higher prices, lower demand
Value of a = 10.13
Value of b = -0.9 à If price is increased by $1, then the demand will fall by 0.9 units
R2 = 0.87 or 87% à it is a metric of how good the regression model is
à varies from 0-1
à higher numbers are better since it shows that the model is able to capture a lot of variation in sales
à Typical threshold for a good regression model is R2 = 0.7 or 70%
à Here, we can say that 87% of the variation in sales is captured by price
Now, we can start making predictions using this regression.
We can first take the prices that are already in our dataset, and compare how our predicted regression line or making predictions from the regression is comparing with actual data. The below chart shows exactly that. We can see that the actual data and the predicted regression line are quite close to each other. This is not surprising, since the R-square of the regression is quite high.
We can also start looking at demand predictions at prices that were not there in the dataset. This way, managers can use regression to make predictions about demand for prices that have not been tested yet.
Once we are able to make predictions, we can move to optimum pricing.